External Projects:
External Projects are opportunities where our UCSF Pepper Center is able to collaborate closely with another institution, organization, or even another department within UCSF to further expand aging-related research. Here are some highlights of our latest work:
Access and Use of Long-term Services and Supports (LTSS) for Older Adults Living Alone with Alzheimer's Disease and Related Dementias from Four Racial/Ethnic Groups
In this study, Dr. Elena Portacolone, Pepper PESC Scholar Alumna, wil be understanding older adults who have Alzheimer’s or similar memory-related conditions and how they live on their own use long-term care services. This is being studied particularly in four different racial/ethnic communities. Working with the Pepper VARC, Dr. Portacolone will create study materials that are easy to read and understand, as well as refine outreach efforts especially with those from communities that have historically not been well-represented in research, along with their caregivers.
Predicting long-term cognitive outcomes and Alzheimer’s disease and related dementias after major noncardiac surgery for older adults
In this study, Dr. Elizabeth Whitlock, Pepper REC Scholar Alumna, will be aiming to understand how likely it is that older adults will experience changes in their memory or develop dementia, and whether they will be able to manage their own finances and medications two years after major surgery. The goal is to use this information to improve care for older adults undergoing surgery. Dr. Whitlock will collaborate with the Pepper DAC statistical team on the use of matching and weighting methods to provide appropriate comparison groups, handling of longitudinal survey weights from multiple survey modalities, detailed guidance on prognostic model development for multiple outcomes, and measurement and analytic methods to add outcomes focused on activities of daily living (ADL) and mobility decline.
Medicare Data Project with UCSF School of Medicine
In collaboration with the UCSF School of Medicine, the Data and Analytic Core (DAC) is leading the effort to sample the Medicare Data (20% Sample) to create a resource in aging across the university and to provide expertise, consultation, and guidance to other researchers as they formulate their research questions and methodologies in utilizing this dataset. This collaboration has been instrumental to forward the research work of Pilot and Exploratory Studies Core (PESC) Scholar, Dr. Catherine Chen (Anesthesiology), and Research Education Core (REC) Scholar, Dr. Tasce Bongiovanni (Surgery).
The ARCH Network
Pepper-Center for Vulnerable Populations Liaison and Criminal Justice and Policy Consultant, Dr. Brie Williams received funding for her R24 entitled “The Aging Research in Criminal Justice Health (ARCH) Network.” Through this, she has created a program that has supported pilot studies lead by 12 researchers across 9 states, who are expanding their research portfolios to understand the healthcare needs of older adults in the criminal justice system. Dr. Williams has also launched bimonthly ARCH Works in Progress seminars for these pilot awardees to present their work to members of the ARCH Network. She also provides consultative support as needed to all pilot awardees of her Network as part of our Pepper VARC office hours.
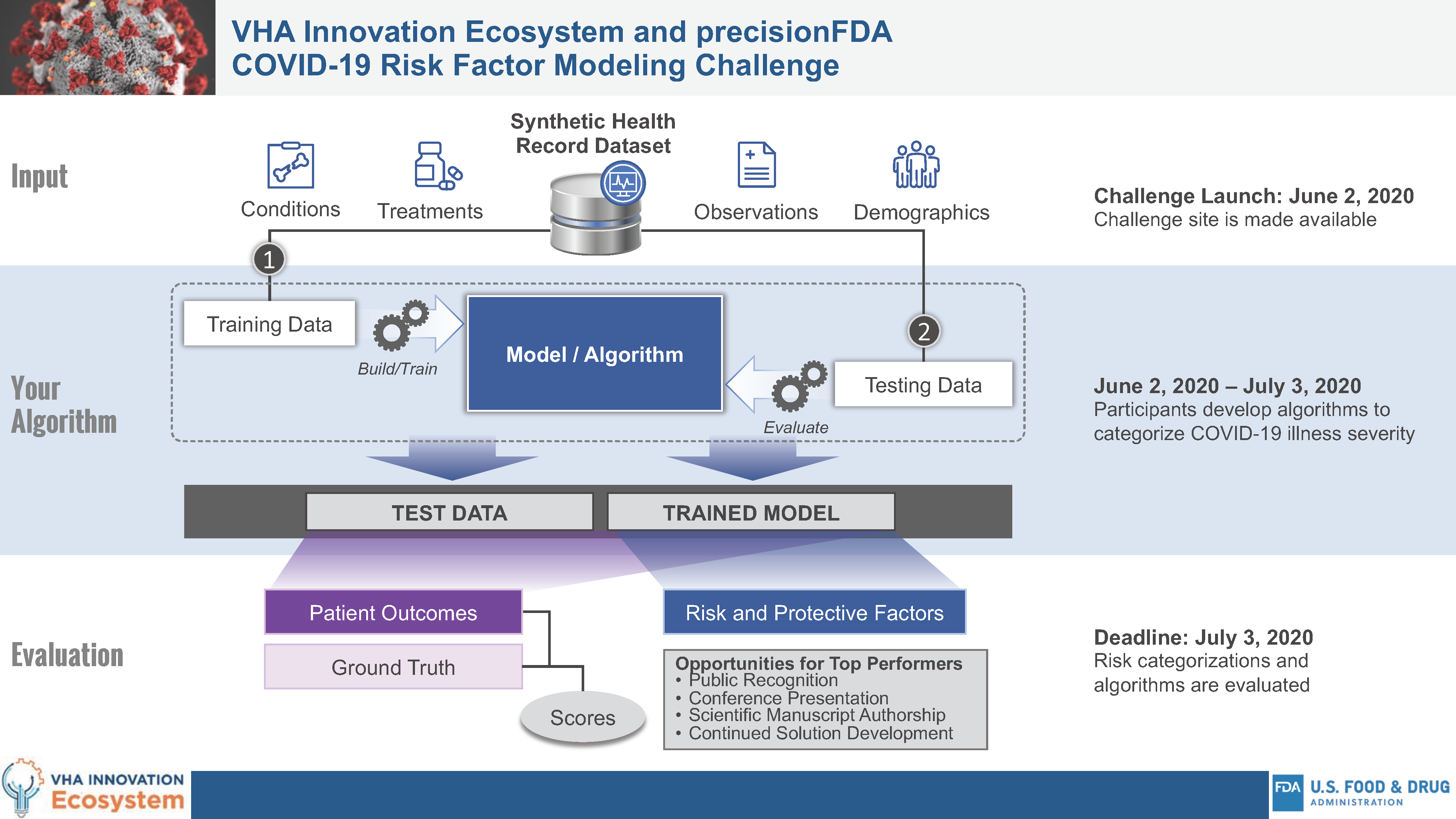
VHA Innovation Ecosystem and PrecisionFDA COVID-19 Risk Factor Modeling Challenge
In June 2020, the Veterans Health Administration (VHA) Innovation Ecosystem and Food and Drug Administration (FDA) called on the scientific and analytics community to develop and evaluate computational models to predict COVID-19 related health outcomes in Veterans. The Data and Analytic Core (DAC) rose to the challenge of creating a model. Their entry was named one of the Three Top Performers. In phase 2, DAC is collaborating with VHA and the FDA to evaluate the efficacy and feasibility of utilizing synthetic data (instead of live Veteran Data) to explore research questions.
Development Projects:
Development Projects are led by our UCSF Pepper Center leaders and scholars where, through their research, they have innovated new scientific developments to enable the progress of their research discoveries. Here are some highlights of our latest work:
Developing an Algorithm to Identify Older Persons with Unmet Need for Equipment in National Datasets
Dr. Kenny Lam (Research Education REC Scholar) and the Data and Analytic Core (DAC) collaborated on developing a novel algorithm that has since resulted in a high-profile publication. The team first approached the development of this algorithm by creating a cohort of older adults aged 65 and above from the nationally representative National Health and Aging Trends Study (NHATS) and selecting participants with bathing and toileting equipment needs. Next, they cross-referenced this cohort with Medicare claims data. Afterward, the team examined how many participants did not receive equipment based on the NHATS annual follow-up interviews, where interviewers meet annually with participants in person to ask about health, function, living environment, and finances and to conduct an objective assessment of physical performance. Lastly, the team used data from the 2016 to 2019 waves to determine the incidence of equipment acquisition among those with unmet needs in 2015. The description of this methodology and the analysis made possible with this novel algorithm has been published in JAMA Internal Medicine.
Deep Natural Language Processing Identifies Variation in Care Preference Documentation
Retrospective chart reviews are one of many methods for researchers and clinicians to extract key information about subjects and patients. However, this is usually a time-intensive process. In the past year, the Vulnerable Populations Aging Research Co-Director, Dr. Rebecca Sudore, and her collaborators have explored the use of natural language processing (NLP) and how it may increase efficiency in performing chart review. NLP (i.e., computer identification of phrases within electronic records) can be combined with deep learning (i.e., computer systems that can access and use information in an adaptive way) to create tools to aid in the rapid identification of care preference documentation. Neural network models are commonly used in deep learning. Similar to the neural networks in the human brain, computational neural networks include a series of statistical algorithms capable of modeling and processing nonlinear relationships between inputs and outputs in parallel and real-time. These algorithms generate rules to associate sequences of words or images on a prespecified concept, such as care preferences, and become more accurate (i.e., learn) with more data over time. This adaptive learning process can be used to abstract complex information from clinical data with an accuracy similar to highly trained humans. As an example of this application, Dr. Sudore and her collaborators have developed and validated deep natural language processing in the identification of documentation of care preferences for patients admitted to the ICU. Their methods and findings can be found in the following manuscript published in the Journal of Pain and Symptom Management.
Methods with Survey Data
There are no straightforward methods or best practice guidelines regarding several important topics in analyzing survey data collected using complex survey designs with weighting, stratification, and clustering. This development project, led by the Data and Analytic Core senior statisticians, Bocheng Jing and Grisel Diaz Ramirez, aims to explore survey data issues from three main aspects: survival prediction (cox model, competing risk), propensity score methods, and linear mixed models.
They have presented their findings at the following conferences:
- SAS Global Forum 2020, entitled "Propensity Score Matching with Survey Data"
- SAS Global Forum 2020, entitled "The Mixed-effects Model and Complex Survey Data"
- American Geriatrics Society 2020 Best Abstract Award in the Epidemiology category, entitled "Population-level cognitive trajectory before and after coronary revascularization in older adults," in collaboration with Drs. Alex Smith (PESC Co-Director), John Boscardin (DAC Co-Director), Ken Covinsky (UCSF Pepper Center Director), Maria Glymour
Barriers and Solutions to Advance Care Planning among Homeless-Experienced Older Adults
Dr. Rebecca Sudore, VARC Co-Director, obtained an R34, with Dr. Margot Kushel, Pepper Pilot and Exploratory Studies Scholar Alumni and Director of the UCSF Center for Vulnerable Populations, to conduct innovative qualitative research to adapt her evidenced-based PREPARE for Your Care advance care planning program for chronically homeless older adults. They have applied the novel Capacity, Opportunity, and Motivation Behavior Change Model (COM-B) to apply and tailor advanced care planning principles to this population, and published their findings in the Journal of Palliative Medicine.
Statistical Harmonization of Two Nationally Representative Data Sets: HRS and NHATS
Through this project, Data and Analytic Core senior statistician, Dr. Sun Jeon is developing harmonized coding of activities of daily living (ADL) and instrumental activities of daily living (IADL) and other functional measures using the Heath and Retirement Study (HRS) and the National Health Aging Trends Study (NHATS). Her work in this field has been instrumental in Dr. Sean Morrison (ISMMS) and Dr. Covinsky's (UCSF Pepper Center Director) P01 project grant titled "Deploying High-Value Longitudinal Population-Based Data in Dementia Research". This work will enable researchers to understand how medical morbidity, social circumstances, and healthcare provision impact outcomes for persons with dementia and their caregivers.
Development of SAS Macros for Logistic and Cox Regression Model Selection
Dr. Boscardin has developed a SAS macro to determine optimism for logistic and Cox regression model selection and fitting for selection methods including the novel Best AIC/Best BIC subset selection methods.
The work was presented at the Global and Regional SAS conferences in 2015 and has resulted in numerous requests from researchers around the world for use of the software. In addition, based on users’ feedback, the Center is revising the macros for re-release.
Specific proceedings papers resulting from this SAS macros include:
- Estimating Harrell's Optimism on Predictive Indices Using Bootstrap Samples (Yinghui Miao, Irena Cenzer, Katharine Kirby, John Boscardin). SAS Global Forum Proceedings, 2013.
Read this article
- Optimism of Best Subset Selection by AIC/BIC for Prognostic Model Building (Yinghui Miao, Irena Cenzer, Katharine Kirby, John Boscardin). Western SAS Users Proceedings, 2013.
Read this article
If you are interested in receiving the macros, please email Dr. Boscardin.